The law that drives the development of AI
Anyone who understands AI's scaling laws understands the future.
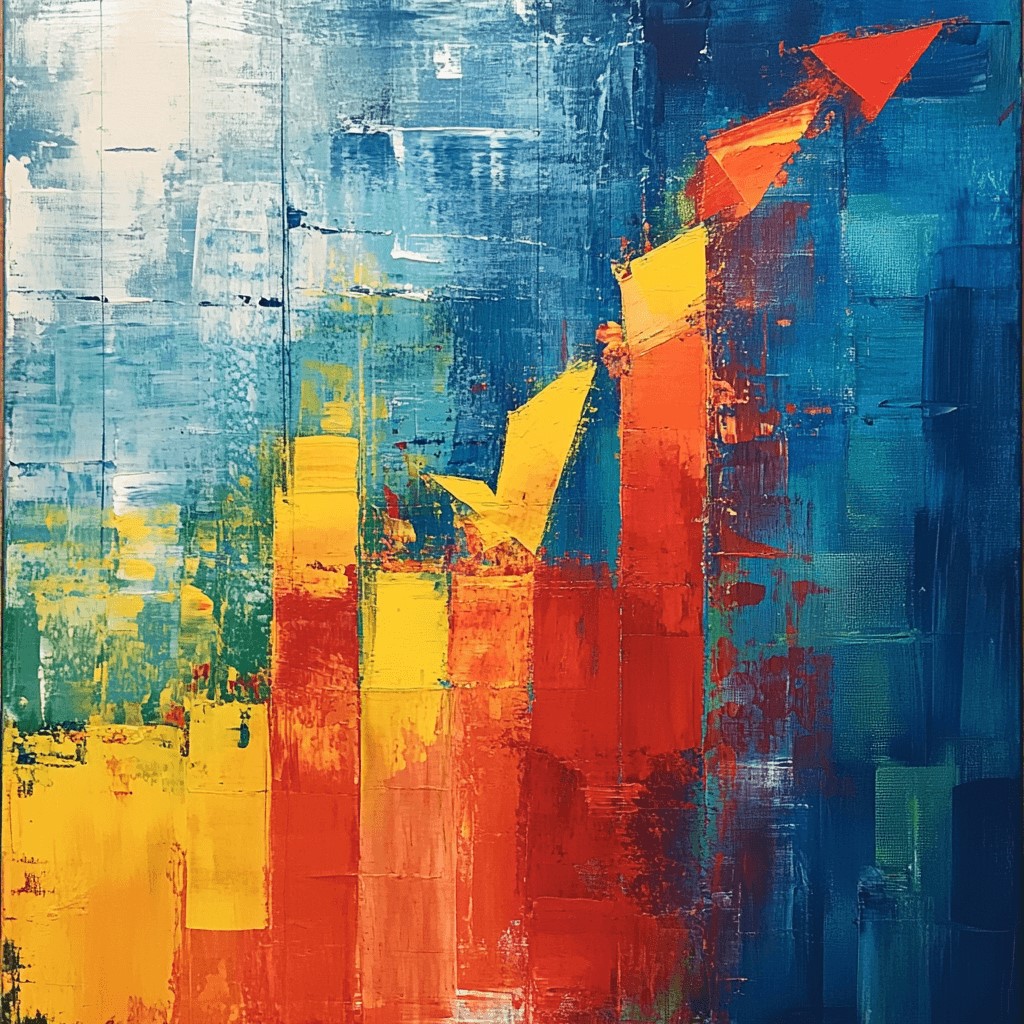
AI-generated illustration from Midjourney
Main moments
When GPT-2, OpenAI's language model, arrived in 2019, no one in Norway raised an eyebrow. The model had major flaws and struggled to solve simple calculation tasks. However, those who were paying attention could see the outlines of something that could revolutionize society, a step on the road towards artificial general intelligence; AI systems capable of performing tasks at the same or better level as humans.
In September 2024, the model GPT-o1 became available on the market. Model now does better on advanced tests in physics, chemistry and biology than people with PhDs in the subjects. Why didn't we see this coming when others did? And where will it stop? There is one thing in particular that separates the far-sighted and those who are taken to bed. It's whether they understand the laws of scaling.
The laws of scaling are the dirty secret of AI development. It's the size that counts. More data and computing power make it possible to train the AI models for longer. And the more they're trained, the better AI systems we'll get. If scaling continues the models will become much more capable in ways that are difficult to predict.
Those who most clearly understood the power of scaling laws were Deep Mind and OpenAI, companies that today lead the development of powerful AI. But even those who develop the systems are surprised seemingly qualitatively new skills. “Until we train the model, it's like a fun guessing game for us [what it might accomplish],” says Sam Altman of OpenAI to the Financial Times about the ongoing training of GPT-5. The development of new AI systems can therefore be experienced as learning to know an alien intelligence while they are made and after they are released on the market.
In Norway, few have caught sight of the power of scaling. It's worse in government. Trygve Slagsvold Vedum rightly made a big deal out of the opportunities in AI when he presented the Perspective Report in August. But did you read the message there were few signs that the government understands the transformative impacts AI can have.
In what is described as an optimistic scenario, economists at the Ministry of Finance estimated that a 10 percent efficiency gain in health care could be achieved by 2060. For comparison estimates thousands of AI experts that it is more likely than not that we will have AI that can perform everyone conceivable work tasks better and cheaper than humans in 2047! Anton Korinek, a leading economist in the field, estimate that “full automation is reached within 20 years”, based on analyses from leading AI experts such as Geoffrey Hinton.
When the government presented its digitalisation strategy at the end of September, there were also few signs that Prime Minister Jonas Gahr Støre and Minister for Digitalisation Karianne Tung understand the laws of scale and the transformative consequences that AI can have.
The new digitisation strategy does not emphasise scaling or the formidable evolution of language models in recent years. When I met Tung for a debate in Dagsnytt 18 she replied that she did not believe in the most severe scenarios as requested by the experts.
What does the Norwegian government know that the world's leading AI researchers haven't realized? There are, admittedly, reefs in the sea. One barrier to continued scaling is that there will be a lack of data. Much of the data available on the internet has already been used to train existing models. And there are big questions about whether AI-created data will be able to improve the models.
Another problem is the lack of computer chips. The value chain that makes the computer chips used in the AI training relies on a very few companies. They may not be able to scale up supply in line with demand.
Nor is it certain that there will be enough power available for further scaling. The more advanced the models become, the more electricity they require, in training as well as in use. Many criticize AI for being an energy sink and a danger to climate and one may wonder if the next “techlashet” will be orchestrated by Greta Thunberg, rather than privacy advocates.
Despite the challenges, there is little reason to believe that we have seen the best AI models. Billions of dollars are invested in the leading technology companies and AI-created data has already been used to improve Anthropic's AI model. Epoch conclusion is that “by 2030, it will with high probability be possible to train models that exceed GPT-4 in scale to the same extent that GPT-4 exceeds GPT-2 in scale”.
What can we expect from such a development? One possible consequence is even greater concentration of power to the tech companies. It will be exponentially more expensive to make new models. Only a few companies can do that.
If the flow of private money stops, it may well be that the states, with their inexhaustible wallets, join in the race for AI supremacy. Maybe it's time for the state's return as an industrial developer?
The states around us understand that in order to manage and be prepared for development, one must examine where the development may go. France, the United Kingdom, the United States and a number of other countries have set up AI institutes, which will, among other things, monitor developments, draw up scenarios, and propose good policies in response to the scenarios.
These initiatives stand in stark contrast to the government's meticulous efforts to increase foresight skills by creating ”a digitalization forum where developments in trends and society at large are discussed annually, including with the parties involved in the labour market”.
No one can predict the future. But what we do know is that whoever doesn't understand the laws of scaling will be helpless in the face of AI developments.